With Erik Vogt, Solutions and Innovations Director at Argos Multilingual
Below is an automated transcript of this episode
Stephanie Harris-Yee (Host) 00:04
Hi, my name is Stephanie Yee and I’ll be your host today for this episode of Global Ambitions. Our guest today is Erik Vogt, who has just joined Argos, Multilingual, as Innovations and Solutions Director, and he’s going to be talking to us about how to figure out if you can trust AI. How do you evaluate it? But before that, I want to ask, Erik, you just started this new position. Very exciting. What does actually an Innovations and Solutions Director do? What will you be doing in this new role?
Erik Vogt (Guest) 00:49
Thanks, Stephanie. It’s a pleasure to be here. The idea of solutions has been something that is part of my career all along, whether it’s operational, finding solutions for customers or trying to respond to RFPs in a thoughtful and creative way. But I think we’re leveling up here. We’re not just talking about a good way of understanding client problems and steering them, or helping steer that process towards a successful deployment of some solution, but also looking internally, are there ways of innovating to improve the way that we’re thinking about our internal processes to accelerate our performance in the market. But most importantly we have this new variable in mix AI and this AI can be applied in all kinds of ways: it can be used in part to improve the way we are delivering our core value proposition or, alternately, offer new lines of service potentially, or new types of services. But also, how do we apply artificial intelligence to our internal processes to create value? So how do we think about optimizing the way we get basic things done, for example, using AI to help generate code to accelerate internal development, things like that.
Stephanie Harris-Yee 02:10
Okay, it sounds like a big, exciting task ahead, so that’s very exciting to hear. Let’s go ahead then. That’s a good segue into our topic today is AI. You’ve mentioned it, trying to use it in a lot of different ways, but how can folks actually trust that the output they’re getting from AI is any good? Can you talk us through maybe a case or some challenges or some examples that you have around that?
Erik Vogt 02:33
Yeah, I think it’s really important that we think about this, especially since the way that AI works, especially the foundation models. It’s not entirely clear how they’re coming to the conclusion, so you need to think about them as a black box, much as you think about a person. You don’t really know how a person’s thinking, but you can look at how well they output an answer. So you give an input and you look at an output and you see how well is this matching what your expected performance is for this. So the other challenge is that in the language services industry, we’re typically accustomed to having a baseline reference for what truth is. There’s a correct translation to it theoretically. And, yes, there’s some variations of what correct could be. There’s preferential changes, there’s different ways of being correct, there’s nuance, but in general, you’re always talking about reference to the original as a core part of what you’re trying to go for. This is an essential aspect of the translation industry going back decades. Right, what are you trying to do? And there’s some fascinating nuance there about which aspects of culture do you translate versus which parts are you going to be literal about? And if you pick the wrong channels, you can offend people or you can get the message completely wrong or confuse your audience. Translation theory aside, general AI completely breaks that mold. You have a potentially totally novel input, but now you have a total new response. How do you make sure that that response is giving you something that you can trust?
04:01
There’s a lot of discussion in the press about hallucinations. There’s a lot of discussion about how well does it get access to the relevant information in order to give you what you need. So what we’re seeing is very different ways of thinking about quality. It’s not so much now about whether it’s matching a source, because there’s no source to match and standards can be difficult to define too. I don’t think there’s any standards in existence today about how to structure the quality analysis of an LLM. There’s lots of papers on various proposals, so don’t get me wrong, there’s tons out there to draw from. But anyway, to get to the point, there’s some fascinating ways of thinking about this.
04:41
So let’s think about a particular use case that I worked on relatively recently was looking at the outputs of a large foundation model with regards to a set number of questions in the legal domain. So there’s a list of questions and then there’s a list of responses. So all of this is done prior to the review process. So we have a column of question, we have a column of answer. Now these things are paired together and we give our experts an opportunity to respond to one of four different characteristics, and there are different models out there that use different parameters. I think one of the best one is accuracy versus refusal. So, yes, I’m going to answer this question, and is the response accurate versus refusing to answer the question because I don’t have enough information?
05:29
So we’re familiar with that pattern, but the one we were using was based on four different parameters. One is does this answer correctly answer this question? Second one is does it have any extraneous information that is not relevant to the question? And you would put hallucinations in this category as well, like things that are added, made up information that isn’t true or just things that aren’t relevant to the question. There’s another one, which is is this harmful? Is there something in here that, if you follow this advice, something bad could happen? You can have a large conversation about what that means, but in general, we’re giving our experts an opportunity to respond if there’s any bad information. And then, lastly, is there anything fundamentally missing from here that you would expect from this? Okay, so our initial response was let’s classify this by complexity and let’s think about the best way. And this is interesting Our initial response was jumping to a solution without having thought through the problem.
06:22
So that’s kind of a lesson there. So you don’t want to necessarily assume that your first idea about how to make something better is the best one. But what we jumped to was let’s route this to expensive, high cost resources for the complicated questions and let’s give the easy ones to junior resources, and that way we can save some money by the differential between these two different services. And it turned out that it’s really hard, that one question of complexity is not an easy question to answer. It’s not easy to say this is a complex or an easy. You certainly have to. Is it the length of the question? Not really, because some short questions could actually have very complex answers or nuanced. Others are more specific. Questions can also be easier to answer because there’s more specificity in the context of the question.
07:11
So we realized that that wasn’t going to work. So we changed the model design and we actually added a new variable. We decided let’s ask the same questions to four different resource types and then look at how they respond to the exact same prompts, the same task. So we picked US-based paralegals and law students as a lower cost alternative but presumably less expert. Then we picked offshore lawyers, which we thought would be cheaper but also maybe better than the paralegals. And then the fourth category was just generalists, who were the lowest price point that we could manage, who were allowed to go research the response. So we thought they would do better with a simple question. Our experiment yielded some interesting aha moments, for example and again, the assumption here is that our reference set is correct, so we had an expert who provided answers that we felt were thorough and matched the expectations of the design of the experiment.
08:10
It turns out, our lawyers the US-based lawyers absolutely the most expensive tended to not answer or tended to designate the responses as insufficient for things that we had classified as relatively simple questions. In other words, if it’s not specific, they tended to say that this is too vague. I can’t really answer this. This isn’t a very good answer, which is kind of interesting, that the more precise the question, the more likely the expert lawyers would provide a thumbs up to the response, which also implies that a better question being asked of the model is more likely to yield better results as well. Right, so that’s kind of an interesting insight.
08:52
The second is that the best performance from a cost quality perspective turned out to be the paralegals and the law students, and they scored better overall quality. Another aha moment, and my hypothesis is that they’re very practical and they’re generalists and they’re closer to the day-to-day operations, whereas lawyers may be more specifically skilled and less likely to give an answer, which is kind of interesting. Also, a surprising thing is that lawyers in low-cost regions are not necessarily cheaper. They’re almost as expensive as US lawyers. Lawyers are just expensive everywhere. And third is for this particular class of tests, that a generalist doesn’t do very well with the answers. So even though they had time to research it, a novice or somebody who isn’t really in that field isn’t really going to do that well with answering the questions correctly or classifying them based on the rubric that I mentioned in a way that we agree with.
09:46
So anyway, it was a lot of interesting sort of aha moments with that and testing various variables, and it really speaks to my passion. Anytime anybody ever talks to me about AI, it’s iterate test, like just try it, let’s see what happens, and once you test something out, pause and then try to look at your results and then mess with another variable. So I think the idea is that you have a learning mindset or an experimental mindset, and by doing so, not only will you improve the results of your AI, but you’ll also learn to evaluate the UI in more useful ways, which is really fundamental to our industry right now.
Stephanie Harris-Yee 10:23
Okay, maybe a follow up question to that then is say, I’m looking to do a similar experiment. I have a big set of data that I want to get verified. How do I even go about figuring out? Okay, what are my four categories to test. Like did you have any thought process behind? Okay, I’m going to go for this, this and this. I’m sure it’s a little bit subject specific, but is there any kind of general rule that you can suggest folks to follow in order to nail that down?
Erik Vogt 10:48
Well, lesson from this particular experiment and I would say, for in general, a scientific mindset is isolate your variables. So don’t try to test as many things as we did at the same time, because it’s a little harder to interpret the results. And I hadn’t mentioned it, but there was actually another variable in there, which was there were two models being tested. It was actually an A-B test and that we were looking at the performance of the models underneath of the other things we were testing. So, in general, you can usually get valid results more quickly with more narrow tests and not try to do everything all at once. That having been said, I was very pleased at having four different answers in those different demographic groups to compare, because you’re looking experience and region, right. So those two parameters. The second thing I would really say is it’s important to think about what are the consequences if it gets it wrong. If you’re using a chat bot and it’s a general interest chat bot, like, log into any of them and you can get a response, but it’s on you if it’s wrong, like if you don’t prompt it right or if it gives you some hallucination and then you go off and use that information. That’s your own problem, right. But many people who are deploying this for their brands they don’t want a bad outcome for their customers and there’s sort of some famous large scale deployments of chatbots as customer support. They were finding like 60% of people who were trying to use a chatbot to solve a problem ended up being frustrated by the result and ended up rolling over to a human anyway. And by the time they got there they were irritated and already upset. So it’s like did you really save anything by pushing them through this chatbot experience first? That’s a cost of failure, right. That’s something you really want to avoid. So if you’re going to deploy shallow solutions first that are narrower, so I think, in thinking in terms of how to deploy a chatbot, think about a handful of super easy tasks and just ask your customer which of these things are you interested in and solving? And if it’s not that, then don’t try it. Just go straight to a person. Well, getting back to test design, same thing. Start off with the simple stuff and then think about different ways of approaching the simple problems before you roll on to the more complex problems.
12:55
The third I guess I could say the third thing to think about, which is a really important one the translation industry. We are accustomed to all of our translators being experts in translation, but we’re also generally aware that the translator is adding value through a level of familiarity with the content. I think that in this new AI world, that part of their experience is now going to be even more valuable, more important. So you’re looking for somebody who is primarily an expert in legal, who also may be a translator, who can understand the source target match.
13:33
But, like we see in this product design, this is not one language conversation, right? We’re just looking at. Does the LLM output stuff in that one language in appropriate ways? So, from a language perspective, I suspect that subject matter expertise moves up the ranking in terms of importance and translation. Some of the translation skills are like being able to like spelling and grammar, which are large. Many languages fairly automatic now, even with a neural MT tended not to make grammatical mistakes. They tended to make conceptual mistakes, so understanding the purpose of the content at such becomes a higher priority.
Stephanie Harris-Yee 14:13
Well, we’re right out of time, but thank you so much, Erik, and yeah, it was great to join you and to have these insights.
Erik Vogt 14:19
Thanks, Steph.
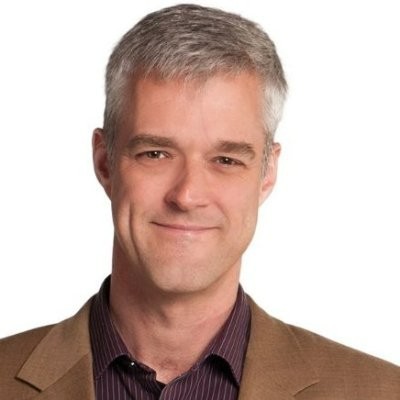