With Chris Phillips, Chief Solutions Officer of Data Services at Argos Multilingual
Below is a full transcript of this episode
Stephanie Harris
Hi, my name is Stephanie Harris, and I’ll be your host today for this episode of Global Ambitions. Our guest today is Chris Phillips and he is the Chief Solutions Officer who heads up data services at Argos Multilingual. And our topic today is going to be a pretty interesting one that I’m sure is on the top of a lot of people’s minds going into the growing field of data services. So, Chris, super glad to have you on the program today.
Chris Phillips
Thank you, Steph. How are you doing?
Stephanie Harris
Doing pretty well. How about yourself?
Chris Phillips
So I’m on the back end of COVID for like the third time.
Stephanie Harris
Oh, no.
Chris Phillips
But look, you know, I’m getting used to it now, as I say, being the third time. But you know what surprises me? It wasn’t so long ago. We’re only talking two years ago. I was flying to Sweden from Munich, and I remember that someone on one of the planes had COVID and they were all taken off the plane by guys in white suits. And the whole plane had to go, you know, go into quarantine.
Stephanie Harris
Oh, my goodness.
Chris Phillips
But thank God those days are over. But yeah, I’m doing good now.
Stephanie Harris
Awesome. Well, I’m glad you’re able to join us today and talk a little bit about data. So this is something that been growing in the industry. We keep on hearing about it in relation to translation and localization, but it doesn’t really fit into that same bucket, right? Can you sort of give a history of how you got started with data as a translation company? How did that even happen?
Chris Phillips
Yeah, well, actually from an individual level within the translation industry, I was exposed to data collection probably around nine years ago and it was just by chance. I had my own company back then. I was specializing in translating into the Nordics and actually one of the big translation companies, similar to what Argos is today, had approached me and said, Look, we’ve just got thousands, hundreds of thousands of words and thousands of sentences that we just need you to translate. And I remember thinking back then, Well, this is easy. Like it’s just random sentences and let’s just get it done. And that’s what we did back then. We just did that, you know?
But as time’s come on, and what I’ve learned today, I actually think what we were exposed to then, the approach was wrong. Now, of course, maybe the requirements weren’t as strict then as they are today. But, you know.
And then how did Argos get involved? Well, in translating for some of the big five, we’ve been working with some of the big five for a couple of years. They had heard that we were delivering good quality translations. They were having some issues with a different vendor. I don’t know the full story, but they came to us and said, Hey guys, can you help us out ? We want to test you out to do some translations for machine learning.
Now, because I’d been exposed to it when we actually saw the job come in, we were able to, you know, adapt the requirements a little bit based on some of the experience I had. And it was relatively small, you know, not as big as what I’d seen before and certainly not as big as what we’re doing today.
Stephanie Harris
Right.
Chris Phillips
And we did a pretty good job. You know, we used the typical translator, editor, quality assurance models that we would use and that worked pretty well for the client. And they kept on coming back. They kept on coming back with smaller chunks. And that’s how we got started, really. And, you know, so much has happened since then.
Stephanie Harris
Yeah. As you mentioned, a lot has happened since then. So have you seen some evolution in how data services works?
Chris Phillips
Definitely so. I mean, if we sort of jump back a little bit as well, it grew so fast and so quick that, you know, at one point we were using traditional translation technology to help our clients get the data collections they needed for machine learning. But then without preparation, suddenly we were told we needed to scale this up to huge levels, 100 plus languages, a thousand plus linguists.
We knew because of the challenges we’ve identified with some of the relatively smaller projects, still big but small in comparison, we knew that the technology was not going to be able to cope with these volumes. So we had to think on our feet and we scoured the market.
We were testing so many different tools to say which one is going to be the best one to handle this volume of people. And we just couldn’t find one wherever they were paid, free, you know, it just wasn’t there. So we ended up coming up with the bright idea that we would develop something, and that is what we we ended up having to do.
Stephanie Harris
Well, it sounds like things are constantly growing and evolving from those first projects, which were pretty straightforward, but then you had to change the methods. So what are you actually doing today with the clients?
Chris Phillips
Today is everything related to NLP, which is natural language processing. So on top of the translation which customers are using to train machine translation models. We’re also doing annotation, search relevance, chat bot, localization labeling, transcription, audio data collection. I mean, the list just goes on and on and on. So anything that’s actually related to human language, we’re involved in helping our clients get data for their machine learning.
Stephanie Harris
So that seems like a lot of diversification with probably a lot of difference between each of these different types. I’m sure there must have been some growing pains to get to that point. Can you share with us what some of those were?
Chris Phillips
Yeah, well, you know, I think the biggest pains we had was diverting too far away from our roots, our roots as a language service provider, because that distracted us. A good example of this is one of our clients wanted audio data collection. Well, okay, we ticked that box. We’ve done that many times, so there’s no problem. The problem was they said we want all the data collection, but it needs to be of guns being fired. And we had to record the audio from these firearms being fired from different distances, so 500 meters, a thousand meters, etc. , etc. . And we took that project on.
Stephanie Harris
Right.
Chris Phillips
It sounds as crazy as it sounds now. I remember having the conversation and I sort of went quiet like, is this for real? And it turned out to actually actually be very real. And we took it on.
Stephanie Harris
Wow.
Chris Phillips
Right. Wow. And I remember coming away from that call, pacing up and down my room thinking, Well, I’ve now said, we’ll do it. How are we going to do it? And we had to get creative. I mean, we had to look around. And the only thing I can guarantee is that no one was hurt and there was no criminality involved in this data collection at all. It turned out we had we were able to get in contact with some people who work with firearms and they were able to set that up and get it recorded. But you see, there was a typical example of us as an organization trying to grow data services as we understood it then, right?
So we felt like, Oh, if we’re going to be successful within the data collection field, we need to take on all types of data collection. And and that was a lesson that we learned that it doesn’t have to be the case. So I think the key thing is trying to find your place within this market.
So if I take the three most common sort of requests that come in to us, we’re looking at sort of. Engineering type jobs. We are looking at language, linguistic NLP, so that sort of fits in the middle. And then we have image and vision. So if we take those three, we will fit very nicely into the middle one, right, Because that’s our roots. We know languages, we work with professional linguists, and then you’ve got the two on the sides.
You’ve got engineering, and then you’ve got image and vision.
Well, from the engineering side, if the scale tips over into that field, we’re okay because historically the language services industry has grown as well using very skilled engineers so we can adapt those.
The challenge comes then when you try to pivot or tilt that scale over to the other side, which is image and vision. In the industry it’s computer vision and for example, annotation in that field and there it gets a little bit challenging. The reason being is, one, it’s either too specialist i.e. labeling MRI scans or x rays within the medical field. Or it requires too large volume at very low cost. So it means you would have to set up production units in very low cost regions, something that, you know, both of these things are doable. We may approach them in the future. It’s just not our primary target today.
So we’re sticking primarily to what we know best, i.e. natural language processing. And we’re certainly diversifying out more into the engineering and field where the engineering and the NLP side are sort of merging together.
Stephanie Harris
Mm hmm. Okay. Well, do you have any examples of what people get wrong or right when they are making these approaches besides, you know, specializing and finding your niche? In the actual work day to day, what do you get wrong or even right?
Chris Phillips
Yeah, I think what we’ve had to learn over the past few years is we’ve got to try and find the balance within the four key areas. Actually, there’s five, but let me focus on the four and I’ll list them out. So I think what you need to do is you’ve got to focus in this order. That’s the scope, tooling, people, quality. And I said there are five, so I’m going to have to include it because it is important. It’s pricing. However pricing, you know, we put that at the end, simply because if you can’t get the other four right, you know,it doesnt matter what, then you can’t figure out the pricing.
So when we take the scope, what we tend to see is we work a lot with data scientists, and data scientists are very good and clear at identifying what it is they want, and they’re very good at that. They know exactly what the outcome should be with this data set so they can train their models.
The problem we’ve seen far too often is that the piece in between the process of getting that data, let’s use annotation as an example, describing how things should be annotated and, more often than not, if you just accept the scope at face value from a data scientist or from the contacts within the organization that is providing you with the work. You could end up with a lot of backwards and forward misunderstandings in what’s supposed to be achieved. So I advise anyone to make sure you go through the scope with a fine tooth comb. Push back if you have to make sure everyone is understanding what it is that needs to be done.
You know, there’s that misconception, and I even had this myself, that if you’re a data scientist, you’ve got to be good with tech and user interfaces of systems, and that isn’t always the case. I mean, of course there are some great geniuses in all fields, but they’re not software people. So that’s where our skill comes in, right? Let’s make sure we understand what it is we need to do.
Once you have that, then it comes on to tooling and what we’ve seen and what we’ve been guilty of this ourselves and we’ve changed the way we do this in recent years is if you try to fit the data set and your workflow into an existing tool, it can lead to delays. It can lead to problems while your people are trying to work within that environment.
So if you don’t get tooling right and it can be very costly as well, right? Because if you’ve got people working in your tools and the tools are not efficient for the type of work that’s being carried out, you end up with too many people dropping off of the projects. The cost of them rehiring, retraining. It outweighs the advantage. So we focus a lot on adapting our tools, making sure we visualize each step that everyone’s going to have to go through while they’re working on the specific data sets that we’re trying to work on.
And then, of course, then comes the people, Right? Right. So the people are key, but there are different levels of people that you need for different types of annotation work. And one we often hear the clients say, Well, we need you to annotate and suddenly, well, what does that mean? Is that just A/B testing? Is this sentence good or bad? Or do you then suddenly need them to be labeling certain words based on grammar rules or any other language type rules? So there’s a huge difference in the skill set required for the people that are working on these projects.
Quality, quality, you’ve got to have a quality strategy before you even get started because you’ve got to know what it is you’re going to measure. Yeah, some projects are easier than others, but in our case, we’ve got data scientists on our side who just help us analyze the throughput and the results of what the people are working on, and that’s coming out with the tooling that we’ve developed.
So coming back to the original question is what do people get right or wrong? Well, if you try to put too much weight on any one of these four areas and not take into consideration or not keep them an equal value, you could run into some problems. We’ve seen it. We’ve learned from that. And that’s what we try to focus on. Find that balance, making sure all of these areas are covered.
Stephanie Harris
Okay, So this is a lot about what’s going on currently, but where do you see the industry going? Things are changing so fast. How do you predict the future for this particular type of service?
Chris Phillips
Well, I mean, the industry is so huge. I mean, the work we do is not even touching the scale of the volumes that are out there and the volumes that are probably coming. Yes, we keep hearing things, you know, It is now 2023, GPT. Everyone’s screaming about it. In my opinion, what it’s doing is it’s creating awareness that people need AI or people can benefit from AI because GPT isn’t the answer to everything. So if the awareness is created and people start realizing that AI can add value to their businesses, well in order for them to use AI or develop machine learning models, they need data. And that’s where companies like us who are working with data collection or annotating, labeling these types of data, we become more in demand. So that’s where I see things going.
Stephanie Harris
Okay. Well, thank you, Chris. This has been very, very informative and I feel like I’ve learned a lot and given a lot of really good insights for folks who are interested in this field and just learning more. So thank you for coming on the show.
Chris Phillips
No, thank you. And if you get any follow up questions or anyone’s interesting about finding out more, please do reach out to me.
Stephanie Harris
Is the best place for folks to reach out to you on LinkedIn.
Chris Phillips
That’s perfect.
Stephanie Harris
Okay, Perfect. Well, thank you.
Chris Phillips
Thank you.
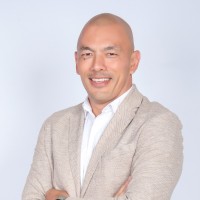